Understanding Machine Learning Data Labeling
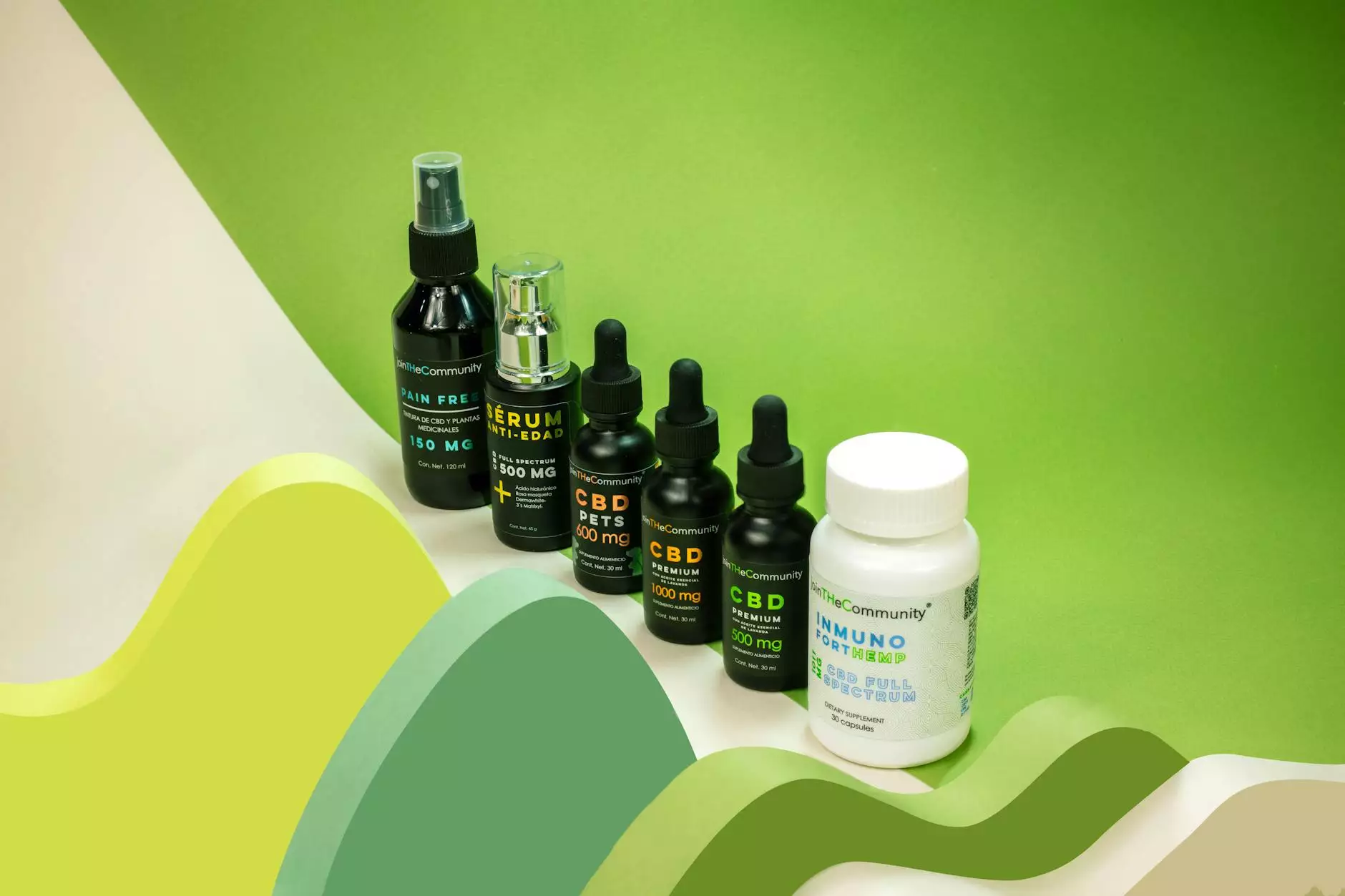
Machine learning data labeling is a critical component in the development and training of artificial intelligence systems. It forms the backbone of AI, enabling algorithms to learn from data effectively. In this comprehensive article, we will delve into what data labeling is, why it matters, and how businesses can leverage data annotation tools and platforms to gain a competitive edge in their respective industries.
What is Data Labeling?
Data labeling is the process of categorizing and tagging data to provide meaningful context that allows machine learning models to learn from it. This process is vital for supervised machine learning models, which rely on labeled datasets to make predictions. During the data labeling process, human annotators assign relevant tags or labels to the data based on predetermined criteria, which can include imagery, text, audio, and more.
Why is Data Labeling Important?
In the landscape of AI and machine learning, data is the fuel that powers intelligent systems. Labeled data enhances the model's understanding and ability to generalize from one situation to another. Here are several reasons why machine learning data labeling is crucial:
- Accuracy: Properly labeled data ensures that the AI can produce accurate results, which is essential for applications such as autonomous driving or medical diagnosis.
- Efficiency: Effective data labeling tools streamline the workload, making it quicker for data scientists and engineers to prepare data sets for training.
- Quality Assurance: Well-defined labeling criteria maintain consistency across data sets, boosting the overall reliability of AI outcomes.
- Scalability: As businesses grow, the ability to scale data annotation processes becomes increasingly important. High-quality data annotations can enhance performance metrics significantly.
The Role of Data Annotation Tools
Data annotation tools are specialized software solutions designed to facilitate the labeling of various data types. They offer a user-friendly interface for annotators and often come equipped with features that enhance productivity and ensure quality. Here’s how these tools can benefit businesses:
Enhanced Productivity
With the right data annotation tool, businesses can experience a significant boost in productivity. These tools often include automation features that reduce the amount of manual labor required. By allowing machine learning practitioners to focus on more complex tasks, data annotation tools make the entire labeling process more efficient.
Collaboration and Workflow Management
Most data annotation platforms include collaboration tools that enable teams to work together seamlessly, facilitating real-time updates and feedback. This capability ensures that all members of a project are on the same page, leading to faster turnaround times and fewer inconsistencies in data labeling.
Quality Control Features
To maintain high standards of accuracy, many data annotation tools come with built-in quality control mechanisms. These features allow organizations to track the performance of individual annotators and implement checks to ensure that labels are applied consistently across the dataset.
Choosing the Right Data Annotation Platform
When selecting a data annotation platform, businesses should consider several key factors to ensure they are making the best choice for their specific needs:
- Ease of Use: Platforms should be intuitive and user-friendly, enabling quick onboarding of annotators.
- Flexibility: The best platforms can handle various data types, including images, video, text, and audio.
- Integration: A good data annotation tool should seamlessly integrate with existing data pipelines and machine learning frameworks.
- Support and Community: Evaluate the level of customer support offered by the platform and the availability of community resources.
Types of Data Annotation
Different types of data require different forms of annotation, and understanding these can help businesses decide on the right strategy. Here are some common types of data annotation techniques:
Image Annotation
Image annotation involves labeling images to identify objects, lines, or boundaries. This type of annotation is essential for training computer vision models. Methods include:
- Bounding Boxes: Drawing rectangles around target objects.
- Semantic Segmentation: Assigning a label to every pixel in the image.
- Polygon Annotation: Using polygons to outline complex shapes.
Text Annotation
Text annotation focuses on labeling various elements within documents, articles, or social media posts. Types of text annotation include:
- Named Entity Recognition (NER): Identifying and labeling proper nouns within the text.
- Sentiment Analysis: Classifying text based on emotional tone.
- Part-of-Speech Tagging: Labeling words with their respective parts of speech.
Audio Annotation
Audio annotation involves tagging audio files for various applications, including speech recognition. This may involve:
- Transcription: Converting spoken language into written text.
- Speaker Diarization: Identifying and labeling different speakers in a conversation.
- Emotion Detection: Analyzing vocal tone to assess emotional states.
Implementation of Machine Learning Data Labeling in Business
The successful implementation of machine learning data labeling can significantly enhance the operational capabilities of a business. Here are some applications across various industries:
Healthcare
In healthcare, labeled datasets can revolutionize diagnostics through the use of AI-assisted technologies. Medical imaging, such as X-rays and MRIs, requires precise image annotation to train models that can predict illnesses from scans. The availability of accurate labeled data helps healthcare providers deliver faster and more accurate diagnoses.
Autonomous Vehicles
For autonomous driving systems, machine learning data labeling is paramount. Labeled datasets provide the necessary context for vehicles to interpret their surroundings. Image annotation techniques, like bounding boxes and segmentation, are critical in helping self-driving cars recognize pedestrians, cyclists, and other vehicles on the road.
E-commerce
In the e-commerce industry, text annotation is crucial for understanding customer sentiment and feedback. By leveraging labeled data, businesses can enhance their recommendation engines, target marketing strategies more effectively, and ultimately improve customer satisfaction.
Challenges of Data Labeling
Despite its significance, data labeling presents certain challenges that businesses need to navigate:
- Labor-Intensive: Data labeling can be a time-consuming and resource-intensive process, particularly when managing large datasets.
- Subjectivity: Certain types of labeling, particularly in text annotation, can be subjective and may lead to inconsistencies if not managed carefully.
- Skill Requirement: Some types of data annotation require specialized knowledge, making it difficult to find qualified annotators.
Future Trends in Data Labeling
As artificial intelligence continues to evolve, so too will the methods and technologies used for data labeling. Emerging trends include:
Automated Data Labeling
With advancements in AI, automated data labeling is becoming more prevalent. Using machine learning models to label data can significantly reduce the time and cost associated with manual labeling. However, human oversight will remain crucial to ensure the quality and accuracy of the labeled data.
Active Learning
Active learning involves training models on a small, labeled dataset and then progressively annotating more data based on the model's uncertainty. This iterative process can optimize the data labeling strategy and improve model performance without extensive labeling efforts.
Integration with Big Data Technologies
As businesses increasingly adopt big data technologies, integrating data labeling processes with these systems will create more streamlined workflows. This integration can enhance the efficiency of data handling and improve the speed at which models can be trained and deployed.
Conclusion
Machine learning data labeling is more than just a necessary step in developing AI models; it is a cornerstone of artificial intelligence that empowers businesses to innovate and stay competitive in an increasingly data-driven world. By harnessing the power of advanced data annotation tools and platforms, enterprises can ensure high-quality datasets that enhance the accuracy and efficiency of their AI systems.
As AI continues to grow and evolve, staying updated on the latest trends in machine learning data labeling becomes imperative. Embracing this strategy not only paves the way for better AI applications but also positions companies at the forefront of technological advancement.
For organizations looking to implement effective data labeling solutions, consider Keylabs.ai as a reliable partner for your data annotation needs